I have many years of experience as a lead architect and SAFe systems architect, with experience in everything from synchronous monoliths to asynchronous microservices. I generally like to work on the technical side of things and I very much enjoy being able to help program the solutions I help to draught. I have accompanied clients through digital transformations, aiding them along the road to scalable agile robust self-healing landscapes.
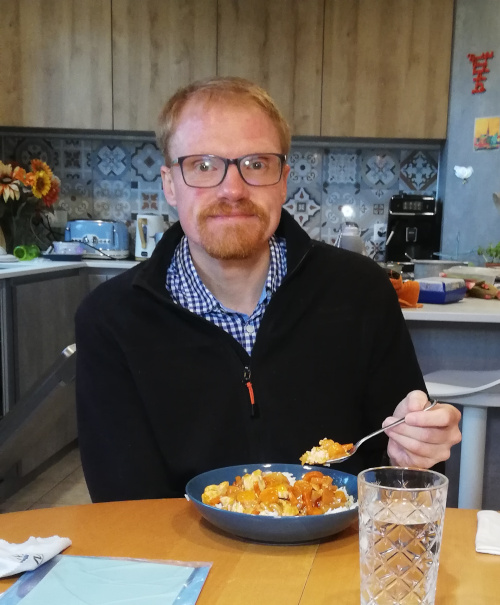
I'm a passionate and partially obsessive software engineer and architect, always looking for a challenge that can be solved with an innovative or pragmatic solution. My aim is to ensure that my customers IT systems are designed, implemented and maintained to a level above and beyond my clients expectation.
Since 2015 I have worked as part of the team at Organit GmbH.